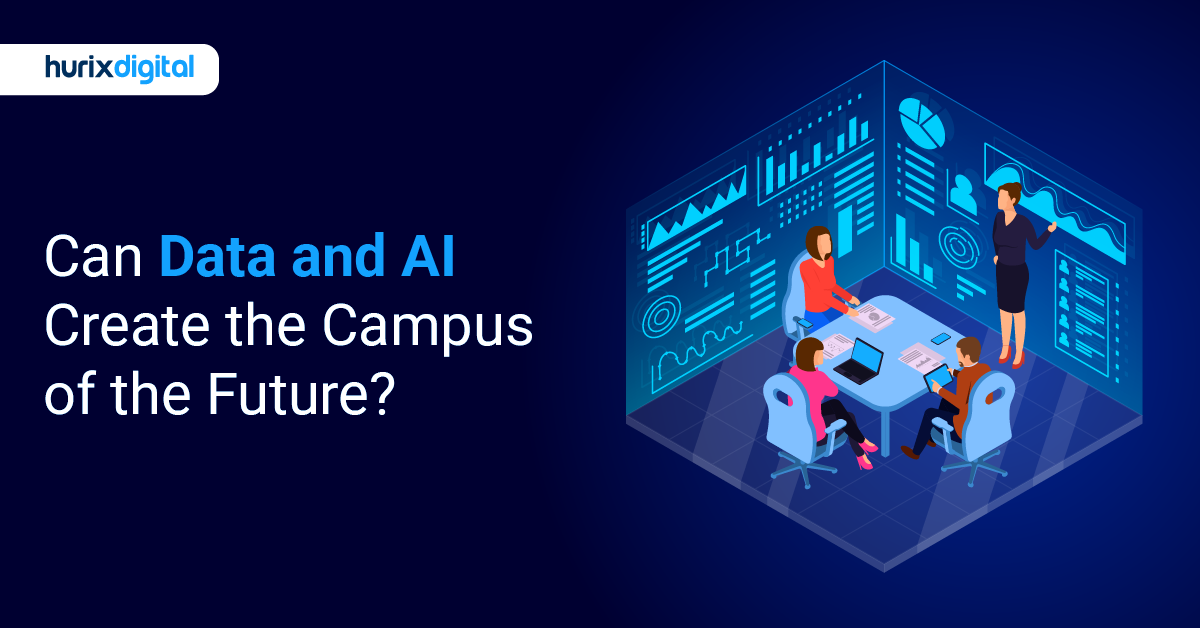
Can Data and AI Create the Campus of the Future?
Summary
This blog examines how data analytics in education can enhance learning, streamline administration, and optimize enrollment, emphasizing AI integration and data-driven decision-making in higher education’s future.
The post-pandemic education era has seen a massive shift toward online learning environments, and the potential of AI to revolutionize education has been recognized. The introduction of AI and Data analytics has produced phenomenal advancements in institutional operations.
The adoption rate of big data analytics and artificial intelligence in enterprises is 60%. As institutions, you realize the need for AI integration in universities for enhanced learning, efficient administration, and quality research.
To keep up with cutting-edge competition, you must understand the complexities of incorporating digital transformation in education and prepare for advanced, future-proof IT infrastructure.
Table of Contents:
- Why Do AI and Data Analytics Integration Matter in Higher Education?
- Understanding Big Data and its Role in Education
- The Benefits of Big Data Analytics in Education
- Why is Data Analytics Important for Student Career Development?
- Developing Strategic IT Plan for AI and Data Analytics Integration
- Investing in Digital Literacy and Professional Development
- Keeping Institutional Goals in Sync with AI Integration
- Cost Factor and Funding
- Preparing for the AI Revolution
- Promoting Interdisciplinary Collaboration
- Addressing Ethical and Security Considerations
- Monitoring, Evaluation, and Continuous Improvement
- Strategies for Integrating Big Data Analytics Solutions
- Understand Big Data Sources
- Use the Right Tools and Big Data Analytics Platforms
- Collaborate with Data Experts and IT Professionals
- Prioritize Data Security and Privacy
- Evaluate Physical Space Utilization
- Promote Continuous Assessment and Iterative Improvement
- Enhance Teaching Methods with Data Insights
- Promote Data Literacy
- Why Data Analytics is Key to Enrollment Success?
- Key Data Points for Effective Enrollment Management
- Mining Your Data Gold: Key Metrics for Enrollment Optimization
- Data-Driven Decisions: From Insights to Actionable Strategies
- Emerging Trends in Data-Driven Enrollment
- To Wrap Up
Why Do AI and Data Analytics Integration Matter in Higher Education?
One of the prime roles of integrating AI and Data Analytics in Education is improving the student’s learning journey, growing the institute’s knowledge base by leveraging the power of AI and optimizing the administrative ecosphere for streamlined decision-making. The following pointers further elaborate on the advantages of AI integration in educational systems and how they impact students, staff, and faculty.
1. Powerful Learning Ecosystems
- Combining Large Management Systems (LMS) with AI in classrooms enables an AI environment that can cater to students’ educational requirements, monitor learning patterns, and craft personalized career paths. This helps break away from the limitations of a traditional classroom.
- Implementing Intelligent Tutoring Systems (ITS) provides students with personalized feedback and learning programs. Your classrooms equipped with ITS can provide timely and targeted assistance to students who can’t reach the faculty.
2. Enhanced Administrative Process
- Leveraging the power of AI and Data Analytics to review faculty performances can help bridge the gap between students and faculty.
- AI chatbots can handle management tasks such as admission processes, financial management, student feedback, and parent queries.
3. Innovation in Research
- Introducing AI research tools in educative programs and using data analytics to back up student research can significantly accelerate research projects.
- Collaborations and interdisciplinary programs experimenting with AI and Data Integration will ensure your institute keeps up with technological advancements.
After understanding the implications of AI technologies in institutes, you must plan strategic advancements to help your institute move towards an AI-enriched ecosphere.
Understanding Big Data and its Role in Education
“Big data” refers to the vast amounts of information generated and collected daily. This data explosion is not limited to Excel spreadsheets and cannot be covered by manual record-keeping.
According to a report, the use of data analytics in education will increase significantly in the coming years. Institutions need big data analytics platforms and advanced big data analytics technology to leverage this data strategically using stringent programs.
Big Data in education includes:
- Student Data
- Course Data
- Instructor Data
- Classroom Facility Data
- Resource Allocation.
Implementing data analytics in education involves several crucial components:
- Data Collection using accurate and reliable data
- Establishing data governance policies to maintain data quality and security.
- Data integration, which combines information from different sources
- Collaborating with data analysts to ensure accuracy.
The Benefits of Big Data Analytics in Education
The global big data analytics in education market is predicted to reach $57.14 billion by 2030, at a CAGR of 15. This growth underscores the increasing importance and potential of big data analytics in shaping the future of education.
Implementing big data analytics in education offers the following benefits:
1. Personalizing Learning Experiences
One key advantage of implementing data analytics strategies to improve student learning is personalizing learning according to each student’s needs. This approach boosts engagement and helps students thrive in competitive environments.
2. Easy Access to Data
With data analytics tools, accessing data becomes streamlined. Big data analytics technology stores, manages, maintains, and organizes information, making it easy for all stakeholders to retrieve the necessary data quickly.
3. Data-Driven Marketing
The marketing teams of various educational institutions use data analytics to create special campaigns that rely on:
- Student Segmentation: Segment students based on academic interests and geographic location.
- Personalized Content: Suggest programs that align with students’ interests and career goals.
- Campaign Performance Analysis: Evaluate the success of marketing campaigns in real time.
4. Data Analytics in Admissions
University admissions teams leverage data analytics in various ways:
- Predictive Modeling: Use historical data to predict a student’s success, considering test scores and extracurricular activities. According to a report in 2022, related to the global education industry, descriptive analytics accounts for 40% of the market share, and predictive analytics accounts for 30% of the market share.
- Application Screening: Speed up the application review process based on a set criterion, along with human evaluation.
- Diversity: Analyze demographic data to address biases in the admissions process.
- Application Feedback: Gather and analyze applicant feedback to improve the admissions process continuously.
5. Data Analytics in Scholarships
Data analytics enhances financial aid distribution:
- Need-Based Scholarships: This helps to assess financial needs based on family income.
- Merit-Based Scholarships: This helps to analyze performance in all fields to award merit-based scholarships.
- Scholarship Package Optimization: This helps to ensure each student receives appropriate financial support.
Why is Data Analytics Important for Student Career Development?
Data analytics plays an increasingly pivotal role in student career development as it provides critical insights that help plan educational and professional pathways.
1. More Effective Guidance
In an increasingly complex and uncertain job market, traditional career pathways fall short and are unable to meet each student’s nuanced and personal needs. Data analytics helps bridge this gap and enhance the effectiveness of career guidance.
One major benefit is its ability to deliver personalized guidance. Data analytics tools can customize recommendations by analyzing students’ academic performance, skills, and interests.
This improves the chance that students will make informed and satisfying career choices. Predictive analytics can also show the growth potential of a particular career option, such as emerging fields in technology and healthcare.
2. Identify Employment Trends
Analytics can help students and universities understand labor market trends and skills that are in demand. Through employment data and alumni information, students can gain real insights into the pathways of particular courses. This enables students to make strategic decisions, ensuring they are prepared for the job market.
3. Placement Programs and Internships
Internship and job placement programs can improve by analyzing patterns in successful placements. Further, feedback and outcome data on internships or placements can contribute to improving career guidance programs.
It can also help placement programs match students with opportunities based on their unique profile, interests, and achievements.
4. Identify Student Strengths
Data analytics can enhance student’s self-awareness by providing insights into their strengths and weaknesses to help them determine which career they are well-suited to. Tools that analyze academic performance, and extracurricular activities, and conduct personality assessments can help identify student strengths and career inclinations.
Developing Strategic IT Plan for AI and Data Analytics Integration
The IT strategies designed to integrate AI and data analytics into education should be tailored to your institute’s priorities and needs. For example, the priorities could include better student learning platforms, avant-research laboratories, or simplifying redundant administrative functions.
An extensive needs assessment can identify your institute’s current IT capabilities, faculty and staff caliber, and student performance for a more informed and prepared digital adoption. Following are some key points to consider:
1. Investing in Digital Literacy and Professional Development
To fully harness the potential of AI and its applications in your institution, you need to train the faculty and staff in digital literacy constantly. Invest in training programs for students and faculty to educate them about AI-driven tools, techniques, and strategies.
For example, Universities like Stanford and MIT hold regular workshops to familiarize their students and faculty with AI and data analytics applications in education, research, and business.
2. Keeping Institutional Goals in Sync with AI Integration
Integrating AI and data analytics in higher education will require an in-depth understanding of what your institution needs and how the AI integration can achieve these requirements.
Defining long-term goals, implementation methodology, data security and management, and stakeholder and faculty development are examples of subtle complexities that are imperative to your institute’s robust planning.
3. Cost Factor and Funding
Once you identify the scale and expanse of AI infrastructure in your institute, review the financial implications. This includes planning for properly architected hardware, software, workshops, training grounds, maintenance costs, and future risks.
Your institute can also actively contact funding sources, alumni, and partnership opportunities to implement the designed digital transformation in education.
4. Preparing for the AI Revolution
Besides stakeholder and faculty engagement, your institute must focus more on student involvement in planning committees and interactive sessions to prepare for the AI revolution.
This engagement enables interdisciplinary innovations, enhanced learning experiences for students and faculty, and an assessment of AI’s practical applications in education ecosystems.
5. Promoting Interdisciplinary Collaboration
MIT’s Schwarzman College of Computing is an excellent example of interdisciplinary collaboration to experiment with the potential of AI and data analytics in education and research.
To ensure constant innovation and growth in educational programs, encourage research projects that bring together experts from various departments to harness the full potential of AI and data integration in education.
6. Addressing Ethical and Security Considerations
Strict security protocols and privacy measures must be implemented to protect sensitive student data and the institute’s information. Implementing ethical AI practices and ensuring transparency in AI decision-making is imperative for the institute’s ethical and moral framework.
Regular security audits, access control, and encryptions are some examples of measures to protect student data and regulate AI on ethical grounds.
7. Monitoring, Evaluation, and Continuous Improvement
Your institute can build Key Performance Indicators (KPIs) to constantly monitor various metrics such as graduation rates, student learning outcomes, teaching practices, research productivity, and operational efficiency. This helps you track the efficiency of your educational IT roadmap and optimize overall digital architecture for a productive AI ecosystem.
Your educational ecosystem must collaborate with industry experts and participate in training curricula to continuously evolve and remain at the forefront of AI evolution.
Strategies for Integrating Big Data Analytics Solutions
Here are some of the integral big data analytics solutions and strategies to improve student learning:
1. Understand Big Data Sources
Finding and understanding the different data sources that are available to your organization is the first step. Important sources of information include demographic data, teacher evaluations, and attendance records.
It is imperative to ensure data accuracy for the following reasons:
- Conducting random checks of individual student performance to validate data.
- Standardizing data collection methods across the institution.
- Comparing qualitative feedback with quantitative data.
2. Use the Right Tools and Big Data Analytics Platforms
Choose the most user-friendly and comprehensive big data analytics platforms for the following benefits:
- Easy to learn for all stakeholders.
- Advanced enough to provide detailed analysis for expert users.
3. Collaborate with Data Experts and IT Professionals
Partner with big data analytics solutions and professionals to develop customized tools for data collection and analysis. This collaboration ensures the tracking of relevant metrics and smooth integration of data analytics into the curriculum.
4. Prioritize Data Security and Privacy
Implement robust security measures by:
- Using strong user authentication and encryption for all kinds of data.
- Implementing strict access controls and keeping software updated.
- Continuously monitoring for unauthorized activities.
- Educating users on security best practices.
5. Evaluate Physical Space Utilization
Optimize the use of physical space by analyzing data on:
- Classroom and resource usage.
- Student movement patterns.
- Course enrollment trends.
- Adjust class schedules and resources to improve efficiency and satisfaction.
6. Promote Continuous Assessment and Iterative Improvement
Continuously assess and improve your data analytics framework by:
- Validating data insights through practice.
- Collecting feedback.
- Using statistical methods to identify flaws in data collection and analysis.
7. Enhance Teaching Methods with Data Insights
Use data analytics to:
- Evaluate the effectiveness of teaching methods.
- Identify areas where students struggle.
- Adjust instructional approaches and content distribution based on data.
For example, if data reveals students are struggling with a concept, teachers can modify their approach or provide additional resources.
8. Promote Data Literacy
It is essential to train both students and faculty in data literacy. Educating them on how to interpret and use data effectively influences informed decision-making. This is effectively based on evidence and not on human intuition.
Why Data Analytics is Key to Enrollment Success?
The world is increasingly data-driven, and any university making the most of the data analytics in education power will compete in the higher education system.
Analyzing huge amounts of data reveals precious information regarding students’ behavior, likes, and trends. Universities’ data-driven approach ensures that such decisions are well-informed for recruitment strategy, campaign marketing, and financial aid.
This benefits the university institutions in optimizing enrollment strategies and data analysis, which includes:
- Revealing Trends: Only through data analysis can a hidden trend and pattern be unveiled. Therefore, you find your student segments with different needs and preferences where you could tailor outreach.
- Predictive Behavior of Students: Using historical data, you can predict which students are most likely to apply, enroll, and thrive at your university. This will help you reach out and target resources accordingly.
- Streamline Registration Process: Understanding bottlenecks and inefficiencies in the registration process through data insights can be adapted to streamline procedures that make registering easier for prospective students.
Key Data Points for Effective Enrollment Management
An application of data analytics in education requires that universities focus first on collecting and analyzing the right points, which can only be achieved by tracking the key metrics from application rates, acceptance rates, yield rates, enrollment trends, and so many others.
Further demographic data regarding academic performance and financial aid utilization will further help unveil student behavior and preferences. To reach your enrollment numbers, gather the right sets of data to analyze:
- Demographic Data: This includes age, location, ethnicity, socioeconomic background, and prior educational experiences.
- Academic Performance Data: Analyze high school transcripts, standardized test scores, and past academic performance.
- Financial Aid Information: Track financial aid applications, awards, and student loan trends.
- Engagement Metrics: Analyze website traffic, application completion rates, social media engagement, and event attendance to understand student interest.
Mining Your Data Gold: Key Metrics for Enrollment Optimization
Maximize the analytics of the data on only a few key metrics that will significantly improve success in enrollment. The average university generates significant amounts of student data. To find actionable insights, focus on the following:
- Recruitment Funnel Analysis: Track student movement through your recruitment channels (e.g., website visits, info sessions). This helps identify areas for improvement and optimize your outreach strategy.
- Application Conversion Rates: Analyze the percentage of students who inquire, apply, and ultimately enroll. Identify what factors influence conversion and leverage that knowledge to boost enrollment.
- Student Retention Statistics: Track student progression and graduation rates to identify opportunities for improvement in learning support services and student engagement initiatives.
Data-Driven Decisions: From Insights to Actionable Strategies
The term “data analytics” in education refers to gathering, evaluating, and applying insights to decision-making. Using data-driven insights, universities can then enhance their marketing initiatives, create a targeted recruitment plan, and enhance the student experience.
This data mining technique improves the effectiveness and efficiency of the enrollment management process by ensuring that every choice is backed up by facts. The institutions will be able to convert their data into useful insights and strategies that can be put into practice.
Better decision-making based on data guarantees that the organizations can do the following:
- Personalizing the Student Journey: Tailor content and communication based on individual student profiles and interests. This creates a more engaging experience and showcases your university’s unique value proposition.
- Finding High-Potential Candidates: Use data to find students who meet your admissions requirements and have a strong track record. Scholarship possibilities and focused outreach are made possible by this.
- Enhancing Advertising Campaigns: Examine the efficacy of various marketing platforms and modify your budget as necessary. Campaigns should target the most likely-to-convert demographics.
- Allocating Resources for Maximum Impact: Based on data analysis, direct resources to projects and activities that have the best chance of increasing enrollment.
Emerging Trends in Data-Driven Enrollment
Under these circumstances, enrollment management is moving toward a more data-driven future. New developments in artificial intelligence, machine learning, and predictive analytics are altering how colleges handle enrollment and recruitment.
With the help of these technologies, institutions can feel considerably better about student behavior and the possibility of future enrollment. The field of data analytics in education is evolving daily.
Consider the following two emerging trends in this regard:
1. Artificial Intelligence Applications
Artificial intelligence is identified as a powerful ally that higher education institutions can exploit to optimize their enrollment process. AI-driven personalized learning contributed to an impressive growth rate of 62% regarding test scores and academic performance.
AI can indeed provide insights through automated procedures and the analysis of huge amounts of data, leading to informed, data-based decisions.
- Student Lead Scoring: With the advancement of AI-based lead-scoring models, students will be scored automatically based on all parameters, including demographics and academic performance. Hence, admissions teams can work with the highest-quality leads and place all the resources correctly.
- Predicting Success Among Students: AI algorithms may scan through historical data and come up with predictive patterns regarding which students might perform better. By pointing out at-risk students early, the institution could support and intervene, eventually enhancing the retention rates for such students.
2. Predictive Analytics for Personalized Learning
Predictive analytics is one of AI’s applications. It bases its predictions on historical data to make projections about the future. Thus, predictive analytics can personalize the learning experience for individual students in higher education.
- Personalized Learning Paths: AI systems will identify each student’s style, rate of progression, and strengths and recommend a personalized path to learning. This can spur enormous levels of student engagement and motivation.
- Early Intervention: Predictive analytics can flag students as likely to fall behind before that happens. Thus, early intervention strategies such as targeted tutoring or academic counseling can keep them on the right track and improve their chances of success. This has proven to raise retention rates amongst students from 3% to 15% respectively.
To Wrap Up
Data analytics in education has emerged as a powerful and strategic tool for universities to optimize enrollment strategies.
Universities can make strategic data-driven decisions while strengthening their outreach and resource utilization, attracting the ultimate student body.
Are you ready to know your power in data and revolutionize how your university approaches enrollment strategy? Hurix Digital can help. With our advanced data-driven solutions, we provide actionable insights and predictive analytics to refine recruitment efforts, target high-potential candidates, and enhance resource allocation.
Talk to us today and explore how our data-driven solutions can help you achieve your goals!
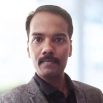
Currently serving as the Vice President of Technology Delivery Operations at HurixDigital, a prominent global provider of digital content and technology solutions for publishers, corporations, and educational institutions. With over 16 years of experience spanning EdTech and various domains, I hold certification as a SCRUM Product Owner (CSPO). My expertise includes operations, finance, and adept people management skills.